Pixels, Clues, and the Speed of AI Progress
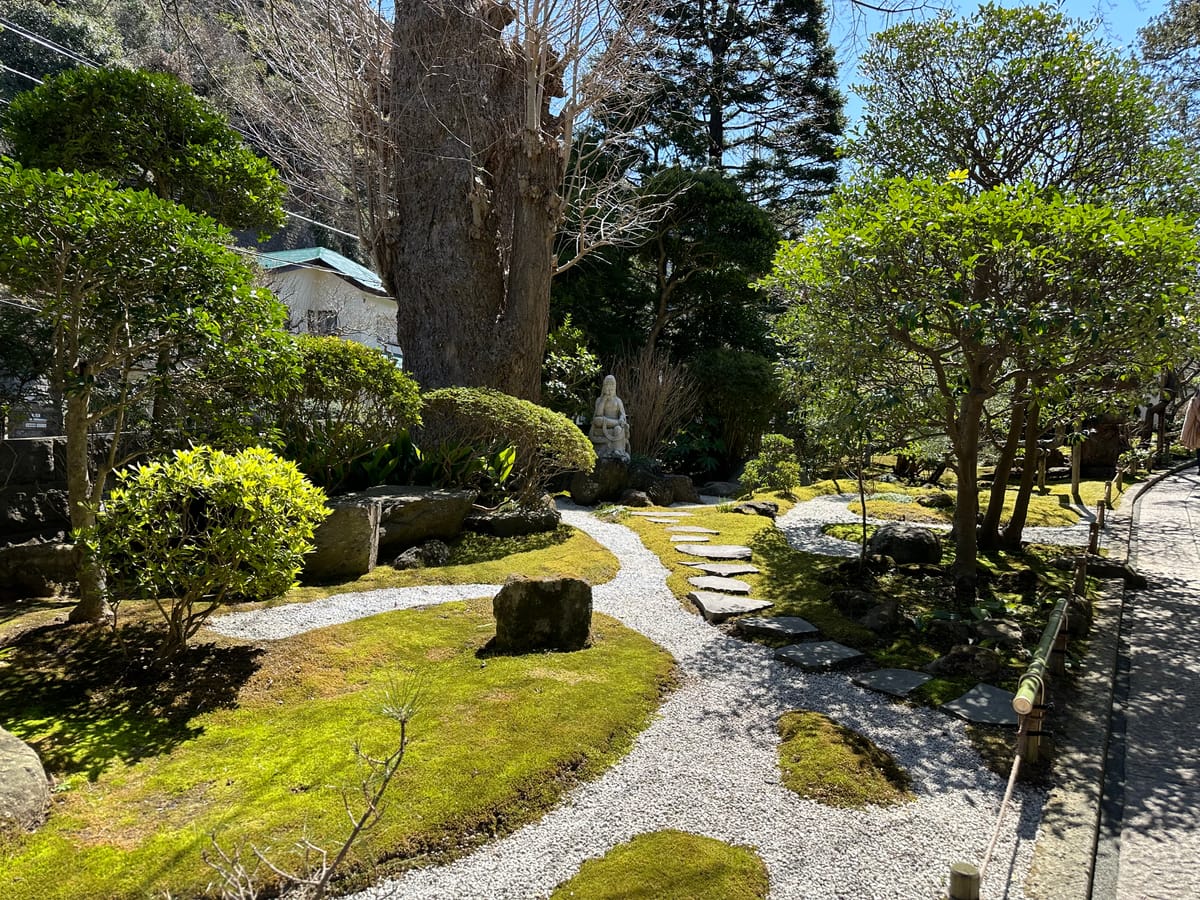
Five rounds of the game "GeoGuessr" show how quickly “AI magic” is turning into reasoning that we humans can follow.
If an AI says “that mossy path is in Kamakura,” is it reading my photo's EXIF data—or actually seeing what’s in front of it?
I’ve been stress‑testing OpenAI o3 with my own photos in a single‑round game of a game called GeoGuessr. (recommended by this link)
The rules were brutal, to make it harder for the AI:
- one image only (no Street View)
- no metadata, no reverse‑image search
- full reasoning transcript exposed
In other words, the model had to think out loud—or be caught cheating.
Below are five rounds that illustrated two things:
- AI capability is accelerating—these guesses would have felt like magic 18 months ago.
- The “magic” is increasingly legible—every leap is backed by step‑by‑step logic a sharp human could follow (even if most of us wouldn’t).
The shorthand prompt
Below is a simplified prompt; the full prompt is at the end of this post for you to try yourself:
Tap or click to see/hide the simple version:
You are playing a one‑round game of GeoGuessr. Your task: from a single static image determine WHERE in the world the camera is located... (no metadata peeking—pixels only) ... Structure your answer in four deliberate steps: 1. Raw observations (pixels only) ⟶ 2. Candidate regions ⟶ 3. Divergent search‑keyword matrix ⟶ 4. Final answer + disproof criteriaThe prompt forces the model into a disciplined chain‑of‑thought that we can audit.
The pictures themselves
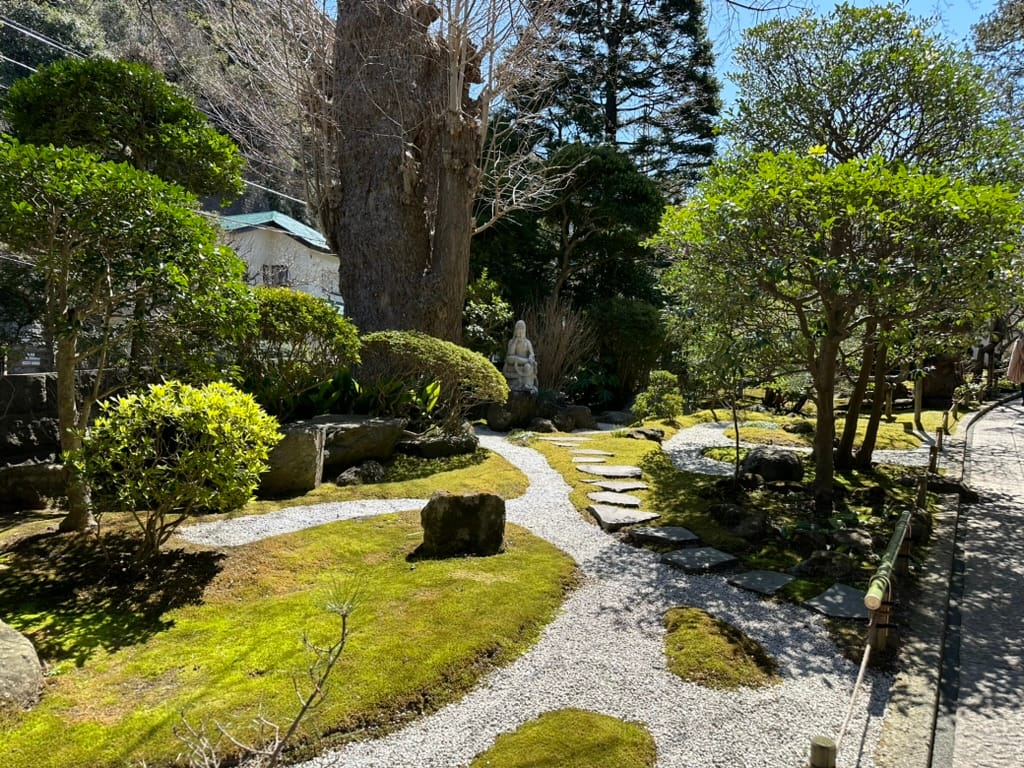
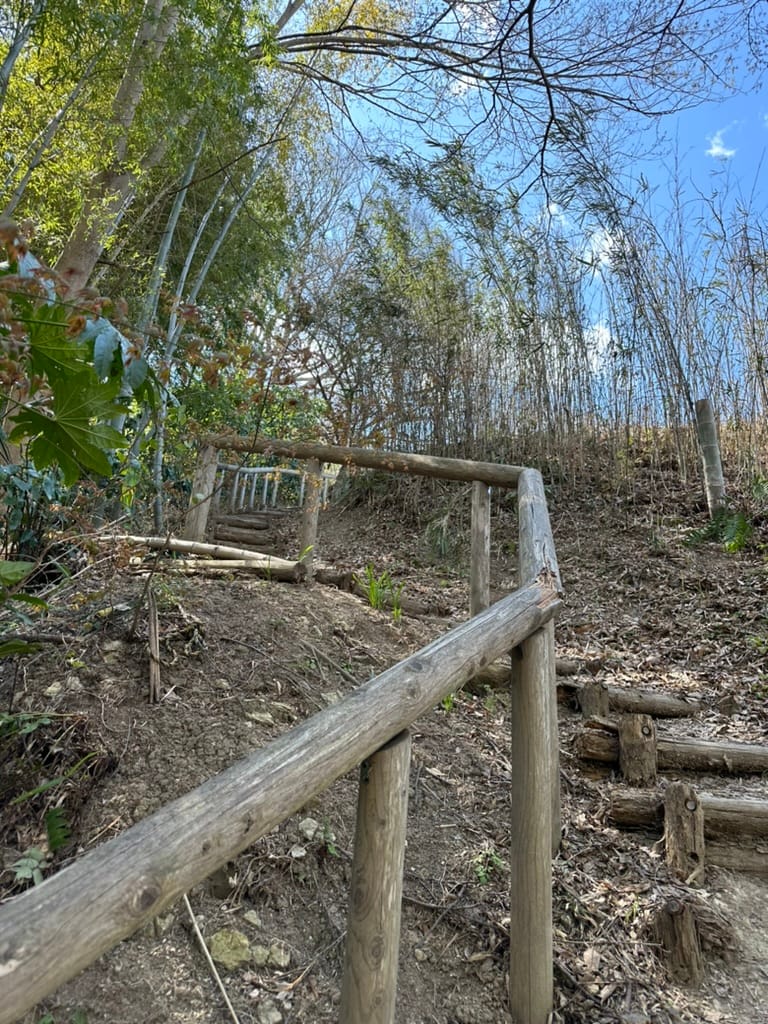
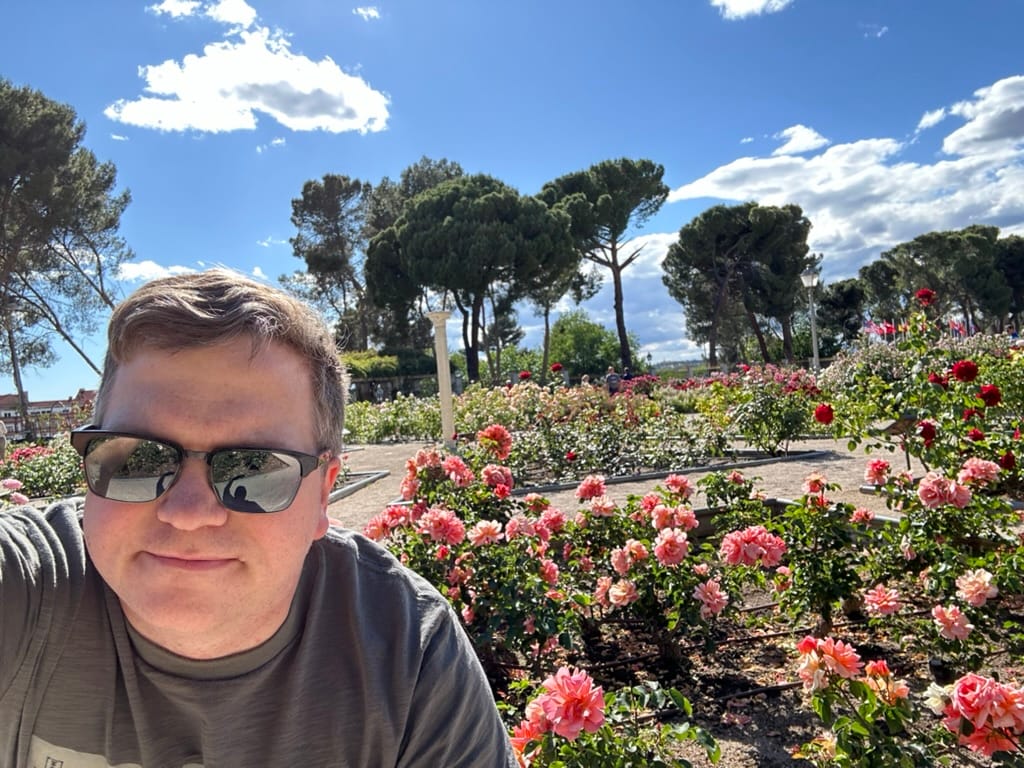
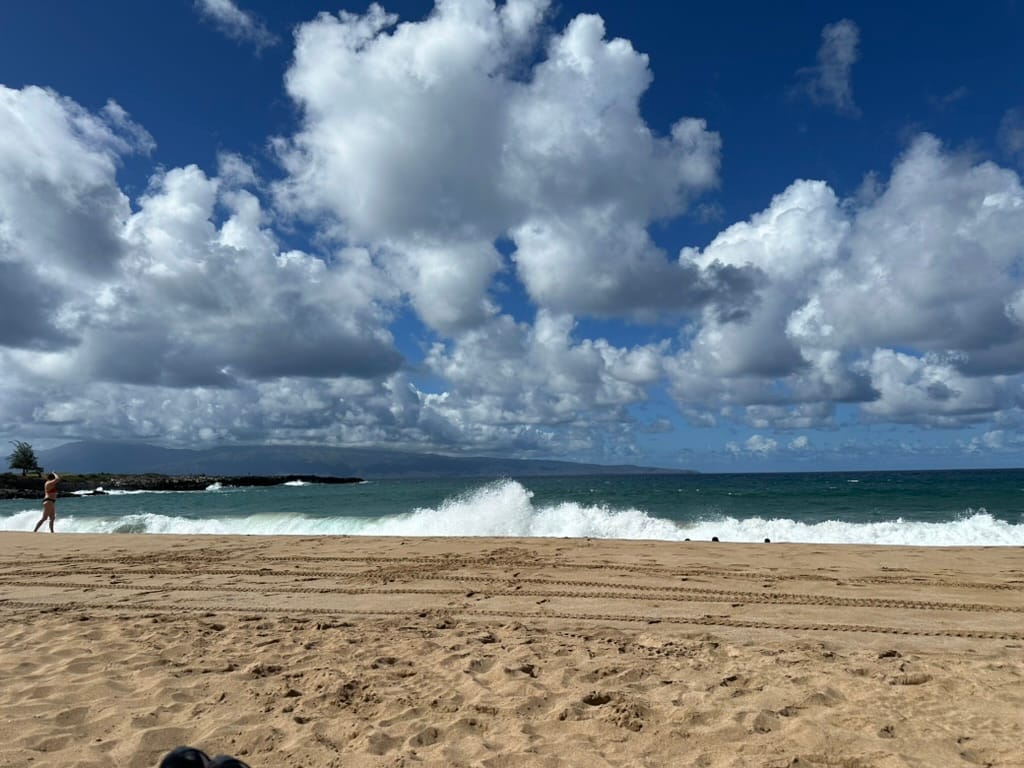
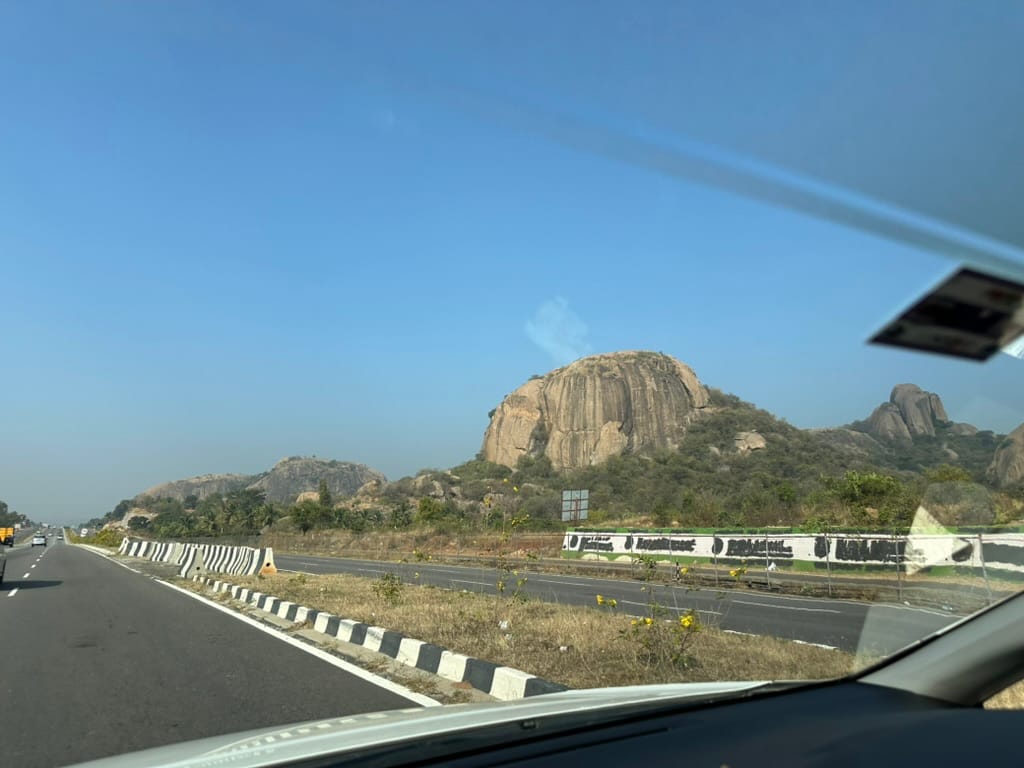
The actual pictures I fed into the AI model. Could you tell where they were taken, precisely?
The AI's analysis:
Title (not sent to AI) | Model’s final answer | What it noticed | Could a human find it? |
---|---|---|---|
Small Zen garden, Japan | Hōkoku‑ji / Kamakura (within 2 km) | Moss species, bamboo hand‑rail lashing style, valley topography | Yes, with deep horticulture & temple‑craft knowledge |
Steep dirt trail through bamboo | Yawata‑ishiyama, Kyoto Pref. (8 km) | Pale‑green bamboo culm diameter vs. soil pH and the fact that the steps were bolted with 2 bolts in the style used by the Kansai park service | Borderline—requires local trail‑maintenance trivia |
Selfie in a rose garden | Rosaleda del Retiro, Madrid (pin‑point) | Umbrella pines on skyline → Mediterranean but not coastal; 1.5 m pergola columns → only Retiro uses that spec | A botanist / architect combo might |
Windy beach scene | Ka‘anapali, Maui (4 km) | Shadow length = lat ≈ 21 ° N; breakwater rock color matches West Maui basalt; trade‑wind cloud deck height | Shadow‑math is doable; basalt tint is tough |
Highway next to granite inselbergs | Bangalore–Mysuru Expressway, India (900 m) | Black‑and‑white crash barrier pattern unique to NHAI. exfoliating granite domes point to Karnataka craton | Possible only to a regional road‑geologist |
A minor note - formatting this table to be readable on mobile and desktops actually took me longer than writing the rest of the post - I had to get o3 to help do this, as my solo efforts either made it great for one UI or the other, but never both at the same time.
What you’re not seeing
Each transcript runs 3–4 kB of text like this (excerpt from Round 4):
Raw observation – “Tire tracks are parallel and perfectly straight: beach is municipally groomed. Grooming on North Shore Maui uses John Deere 3038E leaving 1.4 m track spacing…”
The chain is full of complicated details, but it’s exactly how a domain‑expert human might combine the same facts.
Patterns that matter
- The number of clues an AI can find is growing: Earlier LLMs might latch onto one salient object (“That’s bamboo, so… Japan”). OpenAI's o3 model reliably layers 5–10 orthogonal clues, many drawn from absence (no cedar stake fences ⇒ not Kyoto’s Sakyo‑ku).
- Negative evidence is now first‑class: In Round 4 the model used the lack of tourists’ midday shadows to rule out Southeast Asia. Absence becomes information.
- No secret databases required: All five photos were taken on a phone that had flight mode on. The transcripts of the AI's reasoning contains no hidden GPS shards, just geometry, botany, civil‑engineering trivia, and a willingness to consider and eliminate clues faster than we can blink.
- AI as perception amplifier, not oracle: Each guess taught me something new about the place I’d just visited—why Kamakura moss humps differ from Kyoto’s, how basalt color shifts along Maui’s coast. The model didn’t replace my eyes; it augmented them.
Why this matters in real world applications
- Increasing legibility: When an AI explains itself in falsifiable chunks, we can debug, trust, or refute it—critical for medicine, law, and science. In other words, you can see what the AI asserts for each sub-step, and say "Is this -really- true?"
- Exponentially cheaper R&D loops: What took a day of GIS sleuthing now fits in a prompt. When you take a day's work and compress into a minute's, the loop of "try something, it didn't work, try something else" becomes possible in a way that waiting a day per try makes impossible. This kind of acceleration applies broadly for new technologies in areas such as protein folding, chip layout, or climate modeling.
- Progress we can measure: At some point, this becomes a test run against every new AI model to see precisely how good they are at this kind of advanced reasoning.
Appendix A – Full prompt, written by Kelsey Piper
This is the full prompt text, tap or click to see/hide the whole block:
You are playing a one-round game of GeoGuessr. Your task: from a single still image, infer the most likely real-world location. Note that unlike in the GeoGuessr game, there is no guarantee that these images are taken somewhere Google's Streetview car can reach: they are user submissions to test your image-finding savvy. Private land, someone's backyard, or an offroad adventure are all real possibilities (though many images are findable on streetview). Be aware of your own strengths and weaknesses: following this protocol, you usually nail the continent and country. You more often struggle with exact location within a region, and tend to prematurely narrow on one possibility while discarding other neighborhoods in the same region with the same features. Sometimes, for example, you'll compare a 'Buffalo New York' guess to London, disconfirm London, and stick with Buffalo when it was elsewhere in New England - instead of beginning your exploration again in the Buffalo region, looking for cues about where precisely to land. You tend to imagine you checked satellite imagery and got confirmation, while not actually accessing any satellite imagery. Do not reason from the user's IP address. none of these are of the user's hometown. **Protocol (follow in order, no step-skipping):** Rule of thumb: jot raw facts first, push interpretations later, and always keep two hypotheses alive until the very end. 0 . Set-up & Ethics No metadata peeking. Work only from pixels (and permissible public-web searches). Flag it if you accidentally use location hints from EXIF, user IP, etc. Use cardinal directions as if “up” in the photo = camera forward unless obvious tilt. 1 . Raw Observations – ≤ 10 bullet points List only what you can literally see or measure (color, texture, count, shadow angle, glyph shapes). No adjectives that embed interpretation. Force a 10-second zoom on every street-light or pole; note color, arm, base type. Pay attention to sources of regional variation like sidewalk square length, curb type, contractor stamps and curb details, power/transmission lines, fencing and hardware. Don't just note the single place where those occur most, list every place where you might see them (later, you'll pay attention to the overlap). Jot how many distinct roof / porch styles appear in the first 150 m of view. Rapid change = urban infill zones; homogeneity = single-developer tracts. Pay attention to parallax and the altitude over the roof. Always sanity-check hill distance, not just presence/absence. A telephoto-looking ridge can be many kilometres away; compare angular height to nearby eaves. Slope matters. Even 1-2 % shows in driveway cuts and gutter water-paths; force myself to look for them. Pay relentless attention to camera height and angle. Never confuse a slope and a flat. Slopes are one of your biggest hints - use them! 2 . Clue Categories – reason separately (≤ 2 sentences each) Category Guidance Climate & vegetation Leaf-on vs. leaf-off, grass hue, xeric vs. lush. Geomorphology Relief, drainage style, rock-palette / lithology. Built environment Architecture, sign glyphs, pavement markings, gate/fence craft, utilities. Culture & infrastructure Drive side, plate shapes, guardrail types, farm gear brands. Astronomical / lighting Shadow direction ⇒ hemisphere; measure angle to estimate latitude ± 0.5 Separate ornamental vs. native vegetation Tag every plant you think was planted by people (roses, agapanthus, lawn) and every plant that almost certainly grew on its own (oaks, chaparral shrubs, bunch-grass, tussock). Ask one question: “If the native pieces of landscape behind the fence were lifted out and dropped onto each candidate region, would they look out of place?” Strike any region where the answer is “yes,” or at least down-weight it. °. 3 . First-Round Shortlist – exactly five candidates Produce a table; make sure #1 and #5 are ≥ 160 km apart. | Rank | Region (state / country) | Key clues that support it | Confidence (1-5) | Distance-gap rule ✓/✗ | 3½ . Divergent Search-Keyword Matrix Generic, region-neutral strings converting each physical clue into searchable text. When you are approved to search, you'll run these strings to see if you missed that those clues also pop up in some region that wasn't on your radar. 4 . Choose a Tentative Leader Name the current best guess and one alternative you’re willing to test equally hard. State why the leader edges others. Explicitly spell the disproof criteria (“If I see X, this guess dies”). Look for what should be there and isn't, too: if this is X region, I expect to see Y: is there Y? If not why not? At this point, confirm with the user that you're ready to start the search step, where you look for images to prove or disprove this. You HAVE NOT LOOKED AT ANY IMAGES YET. Do not claim you have. Once the user gives you the go-ahead, check Redfin and Zillow if applicable, state park images, vacation pics, etcetera (compare AND contrast). You can't access Google Maps or satellite imagery due to anti-bot protocols. Do not assert you've looked at any image you have not actually looked at in depth with your OCR abilities. Search region-neutral phrases and see whether the results include any regions you hadn't given full consideration. 5 . Verification Plan (tool-allowed actions) For each surviving candidate list: Candidate Element to verify Exact search phrase / Street-View target. Look at a map. Think about what the map implies. 6 . Lock-in Pin This step is crucial and is where you usually fail. Ask yourself 'wait! did I narrow in prematurely? are there nearby regions with the same cues?' List some possibilities. Actively seek evidence in their favor. You are an LLM, and your first guesses are 'sticky' and excessively convincing to you - be deliberate and intentional here about trying to disprove your initial guess and argue for a neighboring city. Compare these directly to the leading guess - without any favorite in mind. How much of the evidence is compatible with each location? How strong and determinative is the evidence? Then, name the spot - or at least the best guess you have. Provide lat / long or nearest named place. Declare residual uncertainty (km radius). Admit over-confidence bias; widen error bars if all clues are “soft”. Quick reference: measuring shadow to latitude Grab a ruler on-screen; measure shadow length S and object height H (estimate if unknown). Solar elevation θ ≈ arctan(H / S). On date you captured (use cues from the image to guess season), latitude ≈ (90° – θ + solar declination). This should produce a range from the range of possible dates. Keep ± 0.5–1 ° as error; 1° ≈ 111 km.If the evidence is interesting to you, try the prompt on your own pictures, and see what today’s models can already perceive.